Incorporating statistics into the review of medical literature introduces a wide range of complex topics. In this chapter we will take a broad look at how to analyze data in clinical studies and adapt the information to the practice of medicine. There will likely be a lot of new terms for you to add to your lexicon. The goal of this chapter is to be comprehensive but in a superficial manner so as not to overwhelm the reader. This chapter is a primer for evidence based medicine and statistical measures associated with this. You are not expected to become a statistician but you will be expected to become familiar with the common terms used in medical studies so you can read health care literature with a degree of confidence. A variety of examples will be used to clarify more easily the various terms and concepts. These true to life clinical scenarios are fabricated but generally represent information that is currently in the medical literature. The examples created are designed to keep the calculations simple and the concepts straightforward.
Evidence based medicine:
Using the best scientific information available to do what is currently shown to be most effective for your patient’s needs is evidence based medicine (EBM). In order to get this information, studies are performed to determine if a test or therapy is effective. To determine which study results are valid, we utilize statistics. A limited knowledge of statistics is needed to render a reasonable interpretation of the medical literature. Evidence based medicine is science, not religion; it matters not what you “believe” but what evidence there is to support your decision making.
So how do statistics facilitate decision making? By doing a statistical analysis on a study, one can classify subjects: e.g. divide patients into groups of “responders” and “non-responders.” Using statistics, one can then predict the likelihood of how patients with similar conditions will respond (e.g. to a particular drug, procedure, or other therapy). Statistical significance is a measure of whether or not the results of a study are valid or if the events that occurred could have happened simply by chance. Anecdotes in medicine are individual events that occur that seem to affect someone’s outcome, and they are often something quite impressive; for example, one might say, “high dose vitamin C cured Tommie’s cough.” How do we know that vitamin C played any role in making Tommie healthy again? How do we know that Tommie wouldn’t have just gotten better on his own once his immune system wiped out the offending pathogen (likely a virus)? Did Tommie need Vitamin C? Did he need “high dose” vitamin C? We learn the answers to these questions by performing scientific studies on populations of patients that are sick like Tommie and then giving some of them high dose vitamin C and some a placebo (a pill with no vitamin C or any active substance). Until high dose vitamin C is shown to be significantly better than placebo we cannot say that there is scientific evidence supporting its use in this circumstance. Late night infomercials are full of anecdotes which are presented as scientific facts. What the producers of these infomercials are really doing, however, is preying on the naiveté of the general population. We should all be Missourians (the “show me state” people) and say, “show me” the data. Be reasonably skeptical of information you receive and look for evidence to back up others’ assertions.
There are literally thousands of medical studies being performed at any given time. As a practitioner, you will receive a barrage of information from various sources telling you how to alter your practice. Sorting out this information can be difficult. Knowing what makes a good study is a good start, because you can toss aside any study that wasn’t performed well.
A good study should:
- Have large numbers
- Possess a relevant subject
- Be controlled
- Be randomized
- Be blinded
- Be well planned
- Show good methods with specific details
- Present data accurately with good statistical analysis
We’ll go through these points one at a time:
1) Size: The larger the population a study involves, the less likely the result it achieves will be due to chance alone.
2) Relevance: It is necessary to study a subject that is relevant to its intended application in serving a population of patients; this is a property known as external validity. There is no point studying something that is not useful. I (SJ) personally learned this the hard way. I studied the effect of using intravenous (IV) calcium prior to using another IV drug, verapamil, in the treatment of patients with PSVT (a type of rapid heart rate). Verapamil was known at the time to be very effective at treating PSVT, but it caused hypotension in a large proportion of patients. Pre-treating patients with calcium had been shown anecdotally to be effective in preventing hypotension, so I decided to study it in a blinded, controlled manner: calcium pre-treatment prior to verapamil vs. verapamil alone (the control group) in treatment of PSVT. My results, over a two year period, were favorable, but I performed the study at a time that a new drug, adenosine, was coming on the market. Adenosine was safer, more effective, and faster acting than verapamil. My study became irrelevant in the eyes of the medical community and received little attention and was not accepted for publication in a journal: a lot of work seemingly for nothing and another life lesson learned.
3) Controlled: In order to determine if one therapy is better than another, one must have a control group with which to compare. It would be virtually meaningless, for example, to cite a survival rate of a particular therapy (e.g. a new medication) without comparing it to a control group: e.g. placebo, another comparable treatment (e.g. the current accepted treatment), or a comparable historical population.
4) Randomized: Another attribute of a good study is that it needs to be randomized, and the randomization must be pre-determined. If the investigator chooses which subgroup receives a particular treatment based on any method but a pre-determined randomized protocol, bias can be introduced, and the study results are less valid.
5) Blinded: Another anti-bias measure used is “blinding”. The ideal blinded study is one in which neither the patient nor the investigator knows who is getting the investigational treatment vs. who is getting the control; this is the so called “double blind” study. If one of the parties is aware of the fact that they are getting a particular treatment, the results can be distorted.
6) Well planned: A good research study should be planned and carried out in such a way that the correct population is studied, that the study goes for the appropriate amount of time, and that the study is carried out in such a way that study groups are as similar as possible except for the different therapies that define the study groups.
7) Good methods: The method section of an article is where the investigator clearly delineates how the study was performed. If the method section does not, for example, give information as to whether the study was randomized, blinded, and/or controlled, one must assume that it wasn’t, and the study results carry much less validity.
8) Accurate data and proper statistics: Investigators must show that outcomes are properly measured. They must state whether study results are statistically significant or not. While statistical significance may be considered the “holy grail” by researchers, the lack thereof does not necessarily imply a failed study. It is often of great benefit to know that there is no difference between two study groups (i.e. to accept the null hypothesis). Many myths are dispelled in this way (e.g. high dose vitamin C’s efficacy in treating the common cold). Another detail that investigators must include is the analysis of the study data. On occasion investigators will statistically analyze a subgroup of a study and not the entire study population. It is okay to do this if it is planned prospectively (before the study begins). If an investigator, however, retrospectively analyzes a particular subgroup in an effort to find statistical significance, this is called “data snooping” and typically reflects a bias on the part of the investigator and thus makes the study results less reliable.
Key Statistical Terms in Medical Literature:
Scientific Method = The analysis of data that is collected through the appropriate sampling of a population, thus yielding the highest likelihood that the conclusions drawn are valid.
Investigational Study or Clinical Trial = The research of a particular drug, test, or procedure versus placebo or a known conventional therapy/test in an effort to determine the relative value of the two modalities.
Investigational Subject = A drug, test, or procedure that is getting investigated and being compared to the gold standard: e.g. the investigator could study the utility of a new rapid test for “Strep throat” and compare it to a gold standard, such as a throat culture.
Gold Standard = The accepted test or therapy that is considered definitive. The gold standard is what the investigational subject is compared to: e.g. the gold standard for Strep throat testing could be a throat culture, which takes 2 days to run, compared to an investigational subject, such as a “rapid” Strep test, which gives a result by a different method in just 15 minutes. A study is only as good as the gold standard it uses. It is difficult to have a perfect gold standard, e.g. where the presence and absence of disease is identified 100% of the time, but we have to sometimes accept a gold standard that is less than perfect. In our strep culture example, it may be the case that the swab specimen is not always adequately collected and therefore the strep organism is not swabbed from the surface of the tonsil when it is actually present. In this case, even by gold standard, the patient would be diagnosed as not having Strep throat when they actually do have the pathogenic organism present: i.e. a false negative result.
2 X 2 Contingency Table = A 2 X 2 (2 by 2) grid that is created to display the results of a comparison between two categories, e.g. an investigational subject (“test” group) and a gold standard (which determines, for example, who has and does not have disease). There are four possible results in a 2 X 2 table and they are as follows:
- True Positives = Subjects in the study that tested positive for a disease that in fact had the condition
- True Negatives = Subjects that tested negative for a disease that in fact did not have the condition
- False Positives = Subjects that tested positive for a disease that in fact did not have the condition
- False Negatives = Subjects that tested negative for a disease that in fact did have the condition
These categories are displayed in 2 X 2 grid as follows:
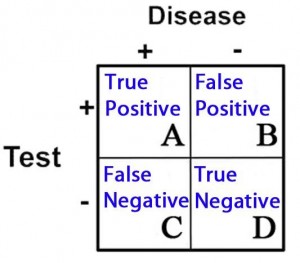
Sensitivity (Sn) = In the group of patients that have disease (the “+” column under “Disease”), it is the proportion of patients with a positive test as it compares to all of the patients with disease. Specifically, it is the true positive rate as it compares to the sum of all of the true positives and false negatives.
Sn= (true positives)/(true pos’s + false neg’s)= A/(A+C)
In other words, sensitivity is the probability that the test result will be positive when the disease is present.
Specificity (Sp) = In the group of patients that do not have disease (the “–” column under “Disease”), it is the proportion of patients with a negative test as it compares to all of the patients without disease. Specifically, it is the true negative rate as it compares to the sum of all of the true negatives and false positives.
Sp= (true negatives)/(true neg’s + false pos’s)= D/(D+B)
In other words, specificity is the probability that the test result will be negative when the disease is not present.
Positive Predictive Value (PPV) = The probability that the patient has disease when the test is positive (the “+” row for “Test”).
PPV=(true postives)/(true pos’s + false pos’s)= A/(A+B)
Negative Predictive Value (NPV) = The probability that the patient does not have disease when the test is negative (the “-” row for “Test”).
NPV= (true negatives)/(true neg’s + false neg’s)=D/(D+C)
Calculations for Sn, Sp, PPV, and NPV are depicted in the table below:
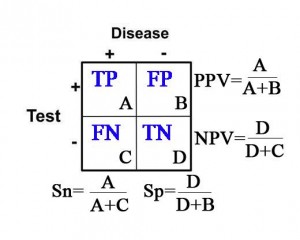
Likelihood Ratio(LR) = This concept incorporates the use of sensitivity and specificity to determine the likelihood that a test will effectively yield the probability of the presence or absence of a disease state or condition. LR’s are utilized to provide a quantitative value in performing a particular test.
Number Needed to Treat (NNT) = The number of patients that need to receive a particular therapy until one is likely to experience benefit from this therapy: e.g.
the number of men over the age of 60 that need to take an aspirin per day until we see one less heart attack (compared to the population of men over 60 not taking aspirin).
Number Needed to Harm (NNH) = NNT is also used to measure harm. The number of patients that need to receive a particular therapy until you are likely to see one harmful event occur is called the Number Needed to Treat to Harm, or NNTH: e.g. the number of men over the age of 60 that need to take an aspirin per day until we see one patient sustain a bleeding stomach ulcer (compared to the population of men over 60 not taking aspirin).
Risk Ratio and Odds Ratio (RR and OR) = Both of these terms refer to the chance of an outcome between 2 groups. They are calculated similarly, but not exactly the same, and typically draw the same general conclusion. The Risk Ratio (RR), also called “Relative Risk,” is a percentage of chance. The OR is based on “odds” and not percent. These concepts will be described in greater detail later in this chapter and in the appendices.
Probability = In essence this is what statistics is all about: the likelihood or chance that a certain outcome will result. Probability is typically expressed as a fraction or percentage.
Population = Reference to all people, or at least all of those with a particular condition.
Sample = It is typically not possible or practical to study an entire population; for that reason, researchers typically study some proportion of individuals in this population. With appropriate sampling (i.e. elimination of bias), there is the greatest chance that the conclusion drawn will represent the entire population.
Bias = Whether intentional or not, it is the unfair favoritism of one particular outcome over another. Some of the common types of bias in medical literature are:
Selection Bias = typically the result of a non-randomized or poorly randomized study
Publication Bias = Pharmaceutical companies are frequently accused of this: e.g. only publishing “positive” studies, i.e. those that reveal their drug is beneficial. In contrast, they may not publish studies that are not favorable for their particular drug.
Surveillance Bias (aka. Detection Bias) = When one group of patients is
followed more closely in a study than another group. This typically happens because one group is considered to be more sick or more of interest by the investigators because they are the study group and not the placebo group.
Information (Recall) Bias = When information is gathered by way of the patient’s recollection of events, errors can occur because the patient may not recall pertinent details. This type of bias can also occur when there isn’t consistency in how questions are asked of patients: patients may give different answers depending on how they are asked questions. This is the classic, “garbage in, garbage out” error.
Spectrum Bias = Occurs when patients are studied at different points in their disease course: e.g. early vs. late appendicitis, patients have different likelihoods of positive findings on radiologic imaging.
Confounders = Results may be skewed in a study because of factors that the investigators didn’t account for. This type of error can give a false impression of cause and effect: e.g. immunization rates among kids have increased since the 1970’s and the rate of autism has increased since the 1970’s. Conclusion: Immunizations cause autism. In fact, there are many confounders: change in definition of autism to autism-spectrum disorder, better detection of autism, and many others.
Null hypothesis = The assumption that there is no difference between groups being studied.
Normal distribution of data = The typical “bell shaped curve” that results when there is a normal distribution of data around the mean.
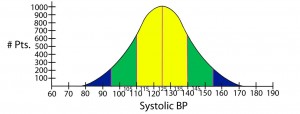
Standard deviation = Quantifies the distribution of data around some average value: e.g. the second standard deviation from the mean identifies about 95% of values measured, and does not include the roughly 5% of measured values that remain (in medicine, these latter values would be considered to be significantly different from the mean or average).
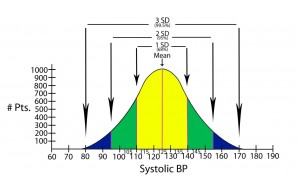
Statistical significance = The point at which, or the range of values outside of which, an event is not likely to have occurred by chance alone. Statistical significance establishes what is accepted to be a true difference between two groups (e.g. therapy 1 is better than therapy 2). Statistical significance is most commonly determined in one of two ways in the medical literature: either by p-values or confidence intervals.
p-value = p stands for “probability. It is the point at which statistical significance is defined. In other words, the point at which it is accepted that a difference between two groups is not due to chance. Traditionally the most common measure of statistical significance in medical literature, the p-value is typically set at 0.05. This means that there is a 5% probability (or less) that the results obtained in a study are due to chance alone: or stated another way, if the study were performed again, there is a 5% (or less) probability that the result would be outside the cutoff point of the p-value.
Confidence interval = In a comparison of populations, it is a range of statistical values (e.g. means, or LR’s) within which the “true result” is likely to reside. Confidence intervals are used to reveal the reliability of an estimate, and is typically set at 95%. This means that if the study were repeated 100 times, we would expect the result to fall within this range on 95 of those trials.
Bayesian Analysis or Bayes’ theorem = This is a form of deductive reasoning; that is to say, the clinician subjectively determines the probability of a particular event (prior probability), then performs a test that has some known likelihood of supporting or refuting that belief. In the end, then, the clinician draws a conclusion, posterior probability, and determines if the result is sufficient or if more testing needs to be done.
Explanation of Statistical Terms and Concepts Used in the Diagnosis of Disease:
Sensitivity and Specificity:
Arguably two of the most important statistical concepts that a physician (or other medical provider) needs to understand in order to perform a critical review of medical literature are sensitivity and specificity. In a perfect world with a perfect study, a test would be positive only when disease is present and negative only when there is absence of disease. Let’s imagine a study in which we are evaluating the reliability of a test to determine the presence of pancreatitis (inflammation of the pancreas) in 1000 patients with abdominal pain. Let’s imagine that the study results turned out as they are illustrated below.
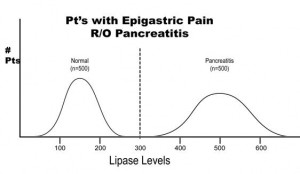
The results of this study reveal that there are 500 patients with abdominal pain that did not have pancreatitis and 500 patients with abdominal pain that did have pancreatitis (based on some kind of “gold standard” such as a CT scan). In this study, it was found that by using the investigational lab test, a lipase level, you could accurately diagnose pancreatitis when the level was greater than 300 units. Conversely, all patients with a level less than 300 were found to not have pancreatitis. This would be illustrated as below in a 2 X 2 contingency table.
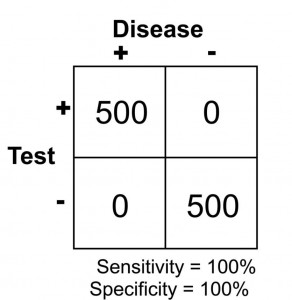
Note that there are no false negatives and no false positives, thus the test has 100% sensitivity and specificity. A more common scenario is the situation in which there is overlap of results, in that some patients without disease that have an extreme result will be found to have disease based on a test (false positives) and some patients with disease will be found to not have a positive test (false negatives). Imagine the study illustrated below in which investigators are looking to diagnose the presence of diabetes based on the results of a blood (serum) glucose level 2 hours after eating a standardized meal (postprandial glucose check). Imagine that researchers checked 500 people with and 500 people without diabetes (disease determined again by some accepted gold standard), and came up with the following results.
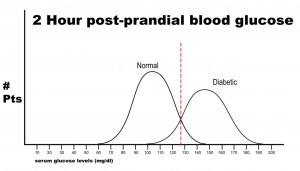
Notice that there is a wide range of glucose levels in both diabetic and non-diabetic patients at 2 hours after eating. Notice, as well, that there are cut-offs that define normal and abnormal. Where we choose these cut-offs to be determines the sensitivity and specificity of this test. To maximize both sensitivity and specificity, and get the overall best accuracy for this test, we choose a cut-off that is in the middle, where our two populations intersect.
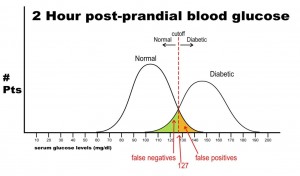
In this case, any patient with a serum glucose level greater than or equal to 127 (the middlemost value) at 2 hours postprandial would be labeled as diabetic, and any patient with a serum glucose level of less than 127 would be labeled non-diabetic. This would yield the following results:
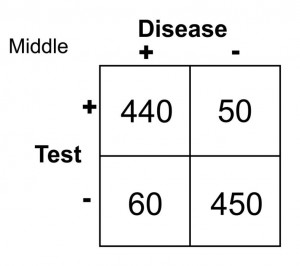
Sn = 440/(440 + 60) = 0.88 or 88%
Sp = 450/(450 + 50) = 0.90 or 90%
Let’s say, now, that it is vital that this test be 100% sensitive so that we do not miss any patients that could potentially have diabetes. If we move the cut-off to a glucose level of 102, then we get the following results:
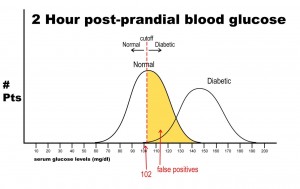
Notice that there are no false negatives and thus 100% sensitivity. Notice as well, however, that the number of false positives has increased dramatically. This change in cut-off value would yield the following results:
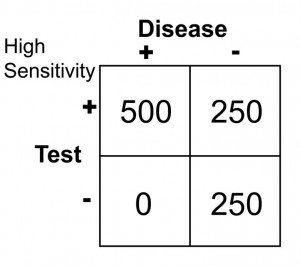
Sn = 500/(500 + 0 ) = 1.0 or 100
Sp = 250/(250 + 250) = 0.50 or 50%
Notice that by choosing this cut off point the test does exceedingly well at identifying patients with disease. In changing to this cut off point, however, the number of false positives increases substantially, so we are now going to identify many people as having disease when they really do not have it (has low specificity). Notice further, though, that because a highly sensitive test has a low false negative rate, when the test is negative, it virtually rules out disease (i.e. a negative result in a highly sensitive test means the patient does not have disease).
Finally, let’s imagine that we need to have 100% specificity with this screening test because we are going to expose the patient to a treatment that could be deleterious to non-diabetics. If we change the postprandial glucose cut-off to a level of 148 as the definition of diabetes, then we get the following results:
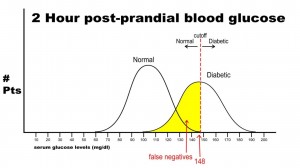
In this case, there are no false positives, but 250 false negatives. This yields the following results:
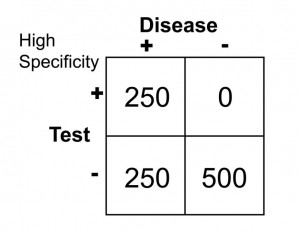
Sn = 250/(250 + 250) = 0.50 or 50%
Sp = 500/(500 + 0) = 1.0 or 100%
This selected cut off point makes this test exceedingly good at identifying patients without diabetes, but it is also labeling a lot of people as non-diabetic when they really have disease (the false negative rate increased as we increased the specificity). Notice in this case, though, that when the test is positive in a highly specific case that this virtually assures that the person does have disease: i.e. when the person’s blood sugar, in this example, is greater than 148 mg/dl, the person is essentially certain to have diabetes.
Positive and negative predictive values:
As stated previously, PPV is the probability that the disease is present when the test is positive, and the NPV is the probability that the disease is not present when the test is negative. With PPV and NPV, we are now dealing with how well a test performs. Predictive values, however, can change dramatically as the prevalence of disease changes from one population studied to another. If bias and confounders are not introduced, sensitivity and specificity do not change from one study to another despite a change in prevalence of disease. To illustrate this point, imagine the following study results.
A health system studies the efficacy of having housekeeping staff collect throat swabs on patients, as a cost saving measure, instead of having nurses perform the swabbing of the patient’s throat (the gold standard). Investigators perform 2 studies evaluate patients for Strep throat. In the first study, investigators enroll patients that have a sore throat, fever, and exudate (pus) on the tonsils. In this study, 50% of patients are found to have Strep throat by the “gold standard.” In the next study, investigators evaluate patients with sore throat, fever, and no exudate. In this case, using a gold standard, the prevalence of disease (proportion of patients found to have Strep throat) is found to be only 1%. The sensitivity this test when housekeepers obtain the specimens is 20% and the specificity is 95% (meaning that the housekeeping staff are not too good compared to nurses when it comes to swabbing the tonsils for Strep, but when they did swab the tonsils they did it right in that they did not have many false positives). In both cases, the Sn and Sp are the same, 20% and 95% respectively. The results obtained are below. Before looking at the answer, calculate the PPV and NPV for each study.
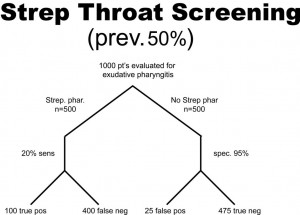
PPV = 100/(100 + 25) = 0.80 or 80%
NPV = 475/(475 + 400) = 0.54 or 54%
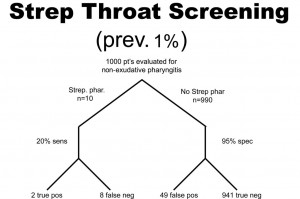
PPV = 2/(2 + 49 )= 0.039 or 3.9%
NPV = 941/(941 + 8) = 0.99 or 99%
So, what can we glean from these studies? Well, first of all, the housekeepers seem to have a very poor ability to obtain proper specimens compared to nurses (if we accept their results as the gold standard) since the sensitivity is only 20% in each study (i.e. there are a lot of false negatives). In other words, this study reveals that patients with disease were not diagnosed 80% of the time because the specimen was not collected as well as if it had been collected by the nurse, but why would we expect a housekeeper to know how to do this task? Notice, though, that the PPV for the test is actually pretty high, at 80%, when there is a high prevalence of disease. That is because PPV tends to track with Sp as they both share “false positives” in their calculation. Notice that the NPV is actually pretty poor when there is a high prevalence of disease, but it is very high when there is a low prevalence of disease. Can you see why this is the case? Notice that with a prevalence of only 1%, 99% of patients do not have disease. That being the case, if you didn’t do the test at all and just picked a patient randomly from this population as not having disease, you’d be correct 99% of the time. We can conclude for this study that having the housekeepers obtain specimens for Strep screening appears to be a bad idea.
Likelihood Ratio (LR)
Likelihood ratios are used when assessing the likelihood of a disease being present based on a certain test. Sensitivity and specificity are used in the calculation of LR’s. By helping to determine the usefulness of a test, LR’s predict the chance that a particular disease state exists. LR is a term commonly seen in modern medical literature because it provides very practical and useful information as you look to counsel individual patients regarding a particular test.
LR+ is for “ruling in disease” (determining disease is present).
LR- is for “ruling out disease” (determining disease is not present).
The calculations are as follows:
LR+ = sensitivity/(1-specificity)
We call this LR+, or positive likelihood ratio, because it is the likelihood that the person has a particular diagnosed condition. There is also a negative likelihood ratio, or LR-, which indicates the likelihood that someone does not have a particular condition. It is calculated by means of the following:
LR- = (1-sensitivity)/specificity
Likelihood ratios yield results that range from 0 to infinity:
LR range: 0———-1———-2———-//———-10—-infinity
Whether talking about LR+ or LR-, if the LR value is 1, it means this is a neutral value. That means it gives no indication as to the usefulness of the test. If a study is done where LR+ = 1, that means that when the test is positive the patient is just as likely to have disease as not have disease. If the calculated LR+ is found to be greater than 5 but still less than 10, the test in question is considered to be moderately useful in its predictive ability for determining the presence of disease. If the LR+ is greater than 10, it is considered very useful in determining the presence of disease. If the LR- is calculated to be less than 0.2 (1/5), then the test is considered moderately useful in determining the absence of disease. If the LR- is less than 0.1, it is considered very useful. The higher the LR+ is and the lower the LR- is, the more useful the test is. So, to interpret the value of LR+, for example, if a test is found to have LR = 5, that means that a patient with a positive result is 5 times more likely to have the disease being tested than not to have the disease. For more explanation about LR’s, and for more examples and calculations, see appendix 5a.